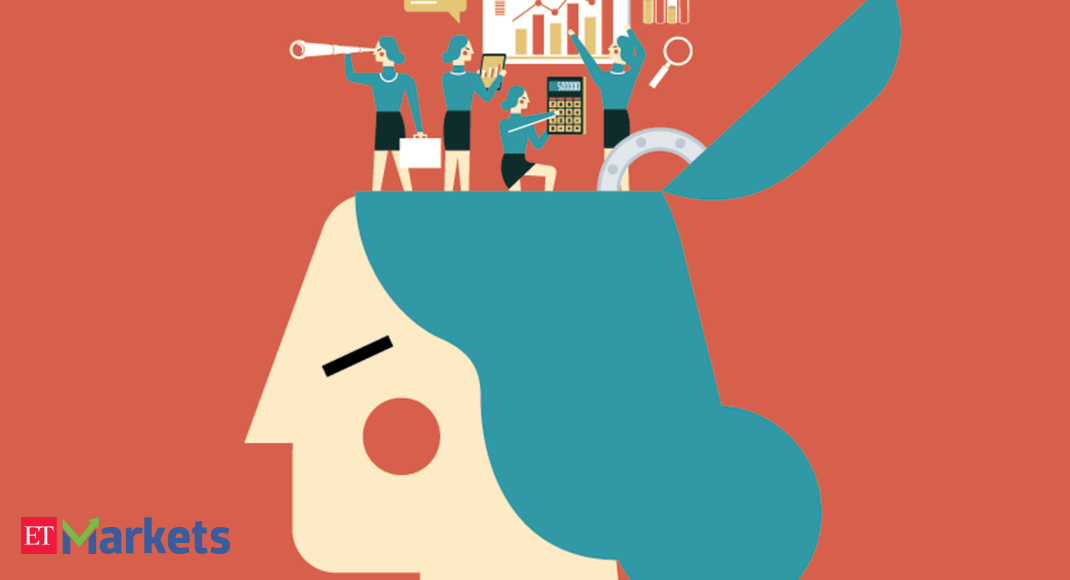
The recent market correction amid the Covid -19 pandemic has bought attention on quant funds, which have stood out with their relative outperformance against the indices.
Boiled down to basics, quant funds are strategies where investment decisions are arrived at primarily using quantitative methods instead of human judgement. Most quant strategies are extremely computation intensive, requiring vast amounts of historical data to come up with signals that play a part in the overall investment process.
Quant strategies are often the outcomes of researchers testing investment ideas for robustness, consistency and longevity. This testing of ideas is often referred to as back-testing.
A back-test is a simulation used to evaluate how effective an investment strategy might have been had it been used historically.
There are many different types of quant strategies in existence, ranging from simple to complex ones. It is important to understand each strategy and then decide if it is right for you.
While the overall success rate of all existing quant strategies is debatable, the biggest benefit they provide investors is that they are based on a framework of discipline. Well-structured models also have an element of robust risk management associated with them.
- Factor Based: The factor-based investing model targets intuitive investment styles such as value, growth and quality, which have demonstrated long-run excess returns across geographies and market cycles and are academically established sources of returns
- Global macro strategies: Such strategies use macro inputs such as inflation, consumer spending, GDP growth rates and employment data to trade across asset classes and geographies. These strategies attempt to build a set of rules that govern the relationship between economic cycles and asset prices.
- Asset allocation: These strategies invest in different asset classes depending on valuation and other signals. The asset allocation framework aims to provide a smoother risk-return profile to the investors.
- Arbitrage strategies: High turnover strategies that seek to identify short-term mispricing in the market to earn a return higher than the relevant risk-free rate. Such algorithmic strategies can be purely based on statistical arbitrage or could involve some residual risk in case of trading around earnings announcements, share buybacks, analystâs revision etc.
- CTA: Systematic trading strategies that employ proprietary trading signals based on technical (e.g. momentum signals) or volatility to generate returns. These strategies often trade in derivatives to take advantage of short term price movements in the underlying. These are typically high turnover strategies and are only suited for sophisticated clients with higher risk appetite.
What separates good quant strategies from bad ones?
The aim of backtesting is to develop robust or successful models and strategies. A robust investment strategy is one that has the ability to adapt to or withstand changes in the market, and maintain positive performance in live portfolios.
Often, strategies that look great in simulation fail to live up to their promise in live portfolios. There are a number of reasons for such failures, some of which are beyond the control of a quant. But in many cases, such failures are caused by common pitfalls in backtesting methodologies, which can be avoided or controlled.
Separation of training data set & out-of-sample data set
It is fine to build a model using available data, but it is not acceptable to test the model efficacy on the same data set used to build it. The data set used for training or creating the model is termed as âIn-sample dataâ and the data set used for testing the model is termed âout-of-sample dataâ.
Avoid data mining and overfitting
Given the enormous data and computing power available, if you generate and test enough strategies youâll eventually find one that works very well in a back test. Here, the back-tested results could be a function of spurious correlation between input variables as they may not have a fundamentally sound basis to persist. Data mining can be avoided by limiting inputs to the quantitative model only those variables that have an economic basis.
Avoiding look-ahead bias and survivorship bias
Use of data for the back test that was only available publicly at the time of portfolio construction and not limiting data to only surviving companies helps not to inflate back-testing results to levels that cannot be replicated in the future.
Why so much interest in quant strategies?
Investors around the world have become savvier and systematic decision making via quantitative models is becoming increasingly popular in comparison with the unstructured way in which investment decisions are arrived at for traditional investment strategies.
This helps minimise human biases, and makes the process more reliable and repeatable. If an investor is looking for transparent and evidence-based investment strategies that combine the best of passive and active management, then they can do well to consider this class of funds to reach their investment destination.
(Prateek Nigudkar is AVP for Risk & Quantitative Analysis at DSP Investment Managers. View are his own)